Advancing Beyond Short-Term Solar Forecasts [Pt.2]
It is crucial to deepen our understanding of the solar cycle and to predict the onset of solar storms.
A single space weather event can cost Europe alone €15 billion, impacting financial markets, power grids, and more. As our economy becomes increasingly intertwined with space technologies, our vulnerability to space weather effects will only intensify. Therefore, it is crucial to deepen our understanding of the 11-year solar cycle and to improve our ability to predict the onset of solar storms.
Issue 63. Subscribers 9521.
In the first part of this series, we explored how solar storms pose risks to both terrestrial and space infrastructure, leading to issues like power blackouts, GPS errors, increased satellite drag, and the potential for collisions. We also highlighted the organizations and companies focused on predicting space weather. Now, we dive deeper into the science of space weather forecasting, particularly focusing on its accuracy and limitations.
Current Accuracy and Span of Space Weather Forecasts
When predicting solar activity and its effects on space weather, the accuracy varies based on what we're forecasting and the time frame involved. The National Oceanic and Atmospheric Administration’s (NOAA) Space Weather Prediction Center (SWPC) provides us with daily forecasts of geomagnetic activity, looking ahead over the next three days. As opposed to the 20-hour ahead forecast provided by Mission Space that we covered in Pt. 1, these predictions are more probabilistic than deterministic, which reflects the current state of our scientific understanding and heliosphere modeling capabilities.
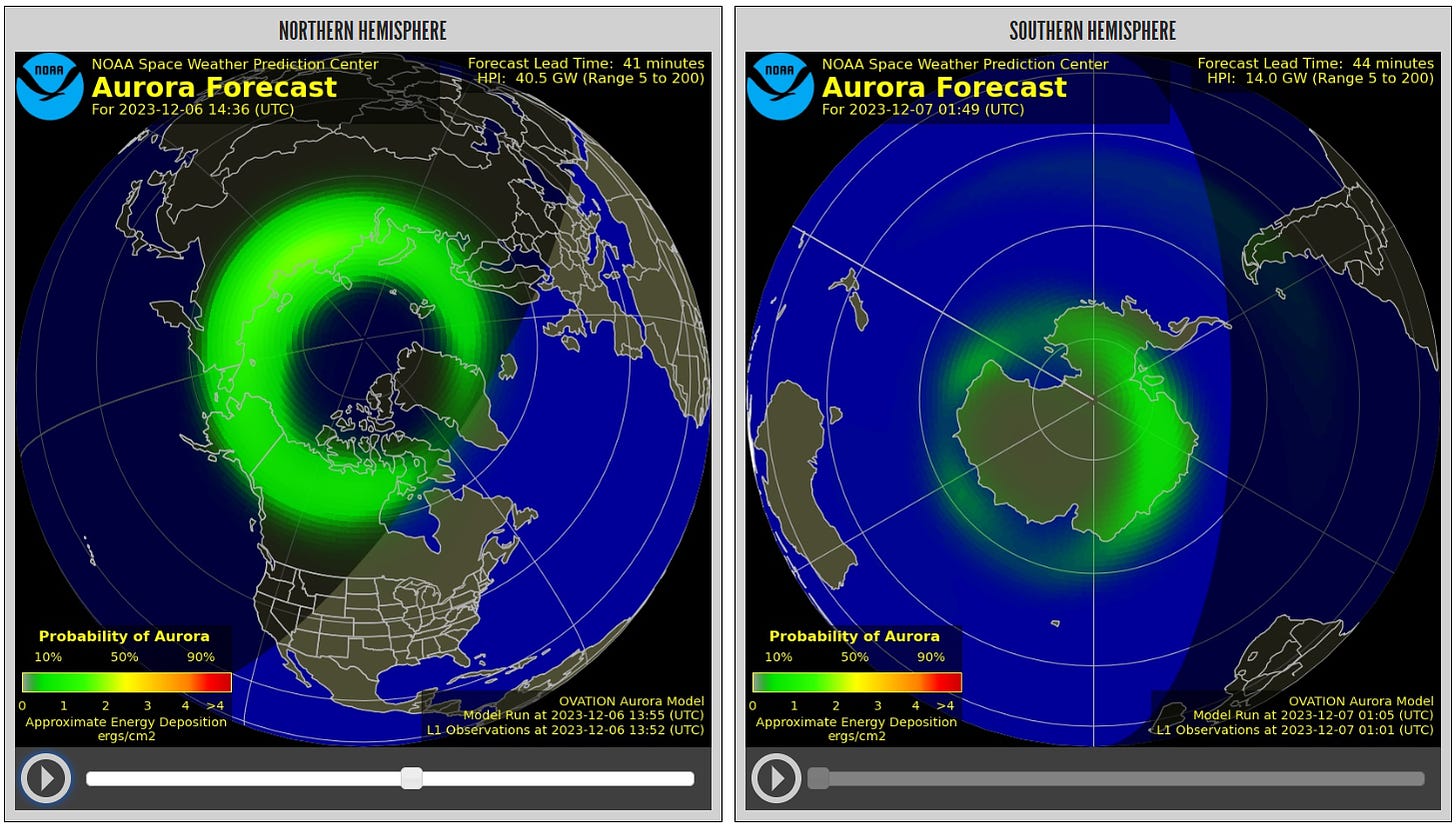
To see why we currently can't forecast beyond three days with high accuracy, it's crucial to understand the mechanics of these predictions. Space weather impacts can be divided into two main categories: radiation and geomagnetic effects. When a solar flare erupts, it releases radiation, including X-rays, electrons, and high-energy protons. X-rays travel at incredible speeds, reaching Earth in just about 8 minutes.
These X-rays (and some highly energetic protons and electrons), alongside visible imagery of the Sun, help us infer that a solar flare has likely triggered a coronal mass ejection (CME). It's important to note that not all solar flares result in CMEs and not all CMEs head toward Earth. However, when a significant CME is directed our way, understanding its trajectory becomes a matter of deterministic plasma dynamics within the Sun's magnetic field. (To put it into a context: near solar maxima, the Sun produces about three CMEs every day, whereas near solar minima, there is about one CME every five days. However, not all CMEs are directed towards the Earth).
The speed of a CME's journey towards Earth varies. Intensive CMEs can arrive in less than a day, while less energetic ones may take up to three days. However, one critical aspect remains beyond our current predictive capabilities: precisely forecasting the moment a solar flare, and consequently a CME, will occur. This limitation underscores the difference between knowing a CME is en route and predicting when one will take place.
Furthermore, NOAA can provide forecasts that span approximately 4-5 years. But it's essential to understand that these longer forecasts are not predictions of specific events like solar storms. Instead, they offer a broader outlook on average solar activity, analogous to climate predictions rather than specific weather forecasts in regular meteorology.
Improving the Forecast Period
Enhancing our solar forecasts is a quite challenging task, primarily due to an incomplete understanding of the physics of Sun’s internal processes. The Sun's complex behaviors, some of which originate deep within its core, remain elusive, as we cannot measure them directly. Solar flares, for instance, are outcomes of the turbulent convective processes closely tied to the Sun’s magnetic field. The solar plasma, highly sensitive to magnetic field, plays a crucial role in these processes.
The Sun's magnetic field, generated by intricate plasma motions, has two components: a dipolar field, resembling a regular magnet, and a toroidal field, akin to those in fusion reactors. These components transform cyclically with 11 year periodicity, which is called an 11-year solar cycle. This cycle is well-observed and studied, yet we still lack a complete understanding of its intricacies.
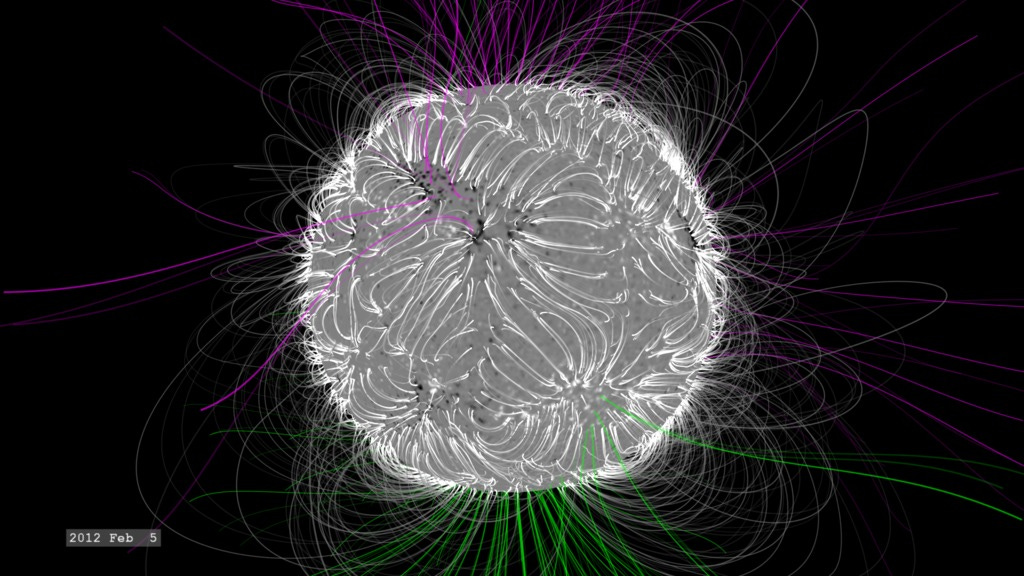
This cycle is driven by the Sun’s dynamic magnetic field. Approximately every 11 years, the Sun’s magnetic poles reverse, marking a phase where the peak in an inner toroidal magnetic field is, leading to increased surface activity and sunspots. As the magnetic field reverts to its polar structure, solar surface activity wanes.
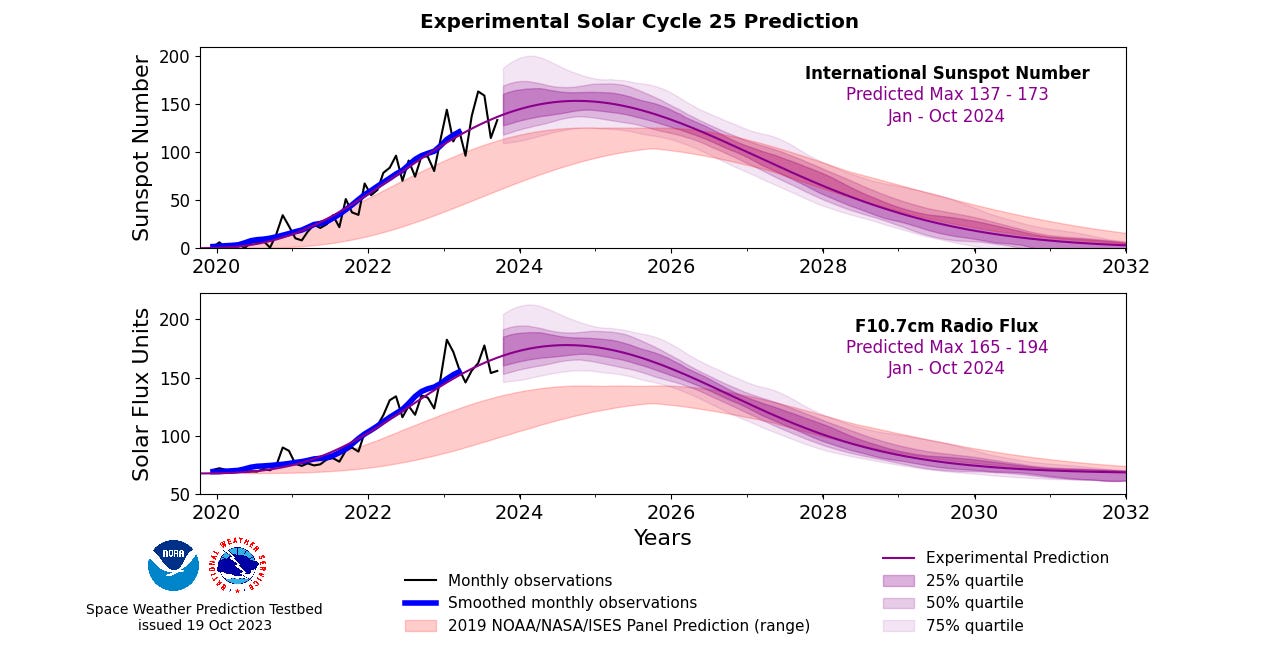
To deepen our understanding of this cycle, several approaches can be employed. Traditionally, sunspot number have been used to gauge the solar cycle, serving as an indirect indicator of magnetic activity. However, newer methods utilize direct observations of magnetic fields on the Sun's surface, leveraging spectroscopic techniques like the Zeeman effect. Combining these surface observations with the Sun’s internal activities data can generate more reliable forecasts than relying on any single data source.
Another innovative approach is applying machine learning (ML). In this context, the precise physics and chemistry underlying solar phenomena need not be completely understood. Instead, finding correlations between the parameters of solar activity, and magnetospheric observations could explain underlying physical processes. Projects like DENSER (deeply understanding space weather) are exploring ML's potential in space weather as well as developing tools for predicting proton flux and geomagnetic activity.
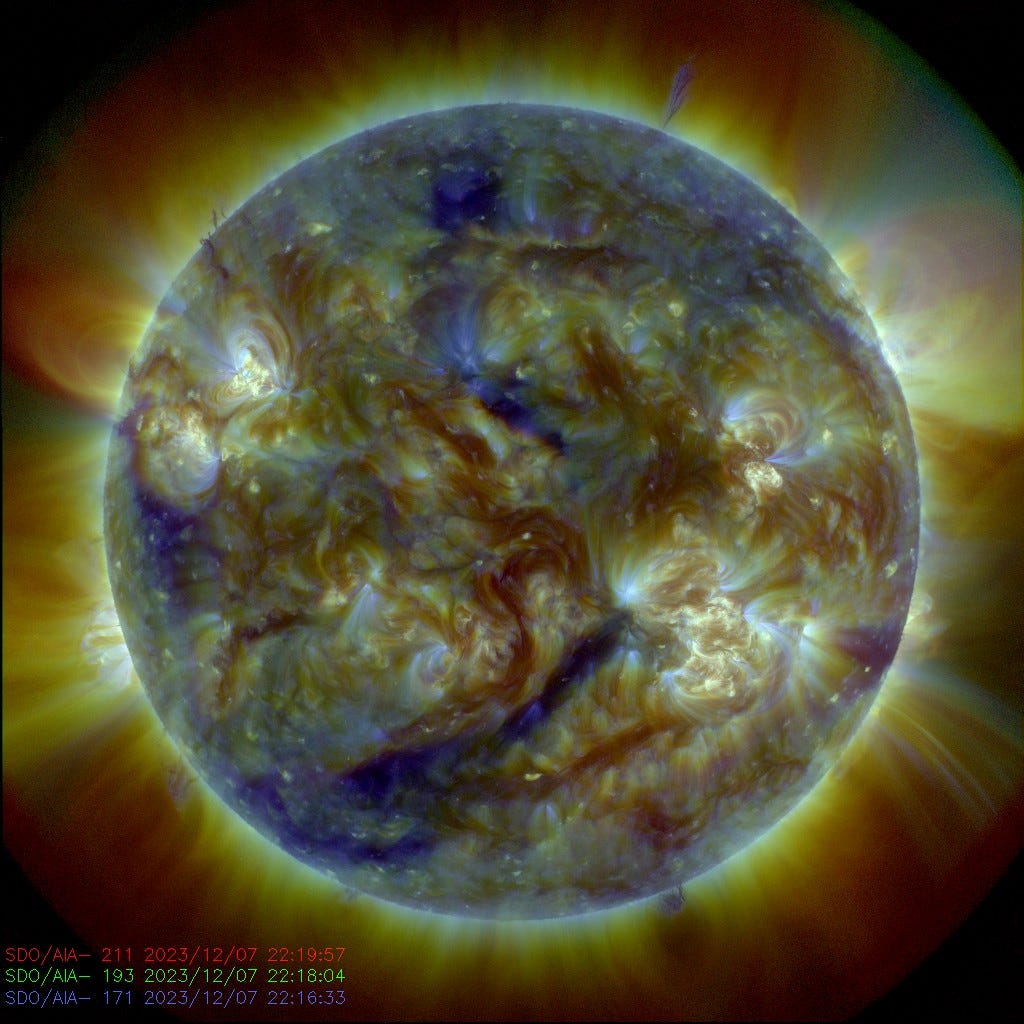
The surge in manuscripts and research employing AI and ML in space weather reflects a growing trend. However, it's crucial to recognize that while ML can significantly enhance our predictive abilities, it is not a cure-all solution. Comparisons with other methods and a thorough discussion of forecast uncertainties are essential to validate these models. Models developed through ML need to be fed with accurate data, validated, and rigorously tested. Sending more spacecraft toward the Sun, like NASA’s Parker Solar Probe will be beneficial. The probe has made history by becoming the first spacecraft to “touch” the Sun. The probe has successfully flown through the Sun’s corona, or upper atmosphere, to sample particles and our star’s magnetic fields. Solar Probe would make direct, on-the-scene measurements right where the Sun energizes its most hazardous solar particles.
Instruments for Better Space Weather Forecasting
The integration of models encompassing solar, terrestrial magnetic fields, and atmospheric data is necessary for enhancing space weather predictions. These models are critical as the interaction between solar wind and Earth's magnetic field ultimately determines the impact of solar storms on our infrastructure.
For several decades, heliophysics has been characterized by acquiring large datasets from sensors in the interplanetary medium, Earth's magnetosphere, and ground-based platforms. A wide array of sensors contributes to space weather forecasts and nowcasts, including:
Telescopes for solar observations in various wavelengths.
Solar Magnetogram Images based on the Zeeman effect.
Solar Radio Bursts: Networks like the International Network of Solar Radio Spectrometers (eCALLISTO).
Cosmic Ray Neutron Flux: Monitored by networks including the Neutron Monitor Database at the Institute for Experimental and Applied Physics, Germany.
Magnetograms at Earth’s Surface: Via networks like the International Real-time Magnetic Observatory Network (INTERMAGNET).
Auroral Visible Imaging: Through systems like the Finnish Meteorological Institute’s real-time auroral cameras.
Ionosphere data: Sourced from the delays of radio signals in the upper atmosphere (Spire Global).
Satellite-based Cherenkov detectors (Mission Space).
Additionally, Japan’s Nagoya University is developing a ground-based, low-cost space weather measurement system using magneto-impedance sensors. This innovation could enable more extensive networks of observation stations, enhancing our understanding of space weather dynamics.
Short-term forecasts, spanning a few hours to three days, become relatively reliable once a CME event has occurred. However, predicting each CME remains a long-term challenge that is still out of our reach. Understanding the solar cycle continues to be one of astrophysics' biggest challenges, with implications not only for space science but for magnetohydrodynamic phenomena across the universe.
A major difficulty is probing the Sun's internal processes, as direct sensor placement inside the Sun is impossible. Here, ML emerges as a promising tool, demanding thorough data collection and analysis.
Considering the socio-economic stakes, a recent ESA study estimated that a single extreme space weather event could have an impact of around €15 billion in Europe alone. As our society and economy become more intertwined with space technologies, particularly satellite navigation systems and space-based internet, our vulnerability to space weather effects will only increase. If we aim to become an interplanetary civilization, overcoming these challenges is essential.